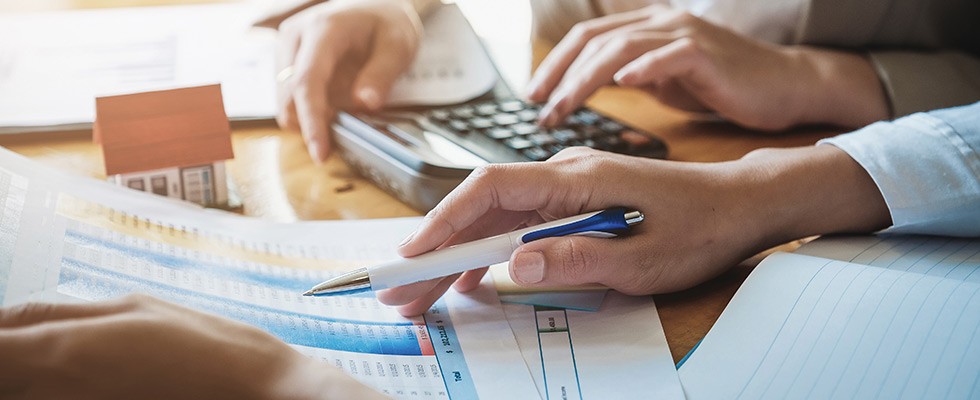
As the aging population grows, home health is experiencing a surge in demand for aging in place and cost-effective care options. At the same time, telemedicine, hospital-at-home programs and remote monitoring are expanding the role of home health in the patient care journey even further—in fact, McKinsey reports up to $265 billion worth of Medicare services could shift into the home this year.
For many post-acute care leaders, these trends all have one clear message: Now is the time to overcome revenue cycle management (RCM) challenges and secure a strong financial position to set your organization up for success in this fast-growing area of health care. Fortunately, data analytics and artificial intelligence (AI) are helping home health agencies optimize their revenue cycle to get further, faster.
The Biggest Challenges in Home Health RCM
A recent survey of more than 400 professionals and leaders across care settings found that 87% of respondents experience significant challenges with claims denials, mainly due to administrative inefficiencies, complex reimbursement models and workforce shortages. While this may not be surprising to home health leaders facing these hurdles every day, the collective impact of these challenges on home health revenue cannot be overstated.
1. Front-End Errors = Back-End Loss
More than half of claims issues begin in the front end of the revenue cycle; of these, 85% are preventable. Front-end RCM challenges can range from coding errors and missing data to lack of prior authorization—but they all add up to a missed payment opportunity, or at the very least, to extra labor dollars spent fixing a denied claim in order to capture the appropriate payment.
For home health organizations, a closer look at the front end could result in significant back-end gains, especially considering that not all denials can be recovered, so proactively addressing common mistakes to lower denial rates can make a noteworthy difference on the bottom line. For example, including prior authorization and a clear summary of benefits in the patient intake process can streamline care delivery, reducing patient care delays while supporting reimbursement efforts for the organization.
2. Complex Reimbursement Models
In the ongoing shift to value-based care from a fee-for-service system, providers must stay on top of new requirements and payment structures, with a particular focus on days of coverage. Coverage ranges vary across payer types; each has a unique set of dates covering a specific set of services, all with their own coding requirements. It’s easy for even the most seasoned post-acute billers to get confused between different payer requirements or to spend hours tracking down the appropriate documentation for services rendered.
This creates a disconnect between providers and payers. Fortunately, some see an opportunity to better align via integrated solutions. With technology keeping billers up to date on the latest payer rules, home health agencies can trust their team is creating claims in accordance with the latest guidance—reducing the frustration many billers face every day and helping the revenue cycle stay on track regardless of payer type, services covered or how long the coverage period is.
3. Nursing Shortages
Just as the growing aging population is increasing demand for home health, an aging workforce and rampant provider burnout are contributing to the nurse shortage across heath care. The number of homecare professionals for every 100 home- and community-based services declined by 11.6% between 2013-2019, with estimates suggesting further decline in more recent years.
In home health, nursing shortages can lead to delayed services, increased workloads for remaining staff and reduced access to specialized care, ultimately affecting care quality and agency capacity. Beyond the revenue cycle, this presents a risk for lower quality scores and outcomes, which can then impact regulatory reimbursement dollars and further impact an organization’s total earnings.
Where AI & Predictive Analytics Make a Difference in RCM
Though the road to revenue cycle success may seem steep for home health leaders, AI-powered technology and predictive RCM analytics offer hope. In the same survey mentioned above, 43% of respondents recognize AI’s potential to reduce workflow burden and enhance efficiency across the revenue cycle.
In the front end of the revenue cycle, AI-powered RCM software can securely reference patient data to confirm patient eligibility in real time. No more bouncing between payer portals or picking up the phone—instead, a quick search matches a patient’s demographics with their active insurance coverage, accelerating access to care and reducing administrative delays at intake by catching errors—and therefore preventing denials.
On the back end, AI helps prevent denials by flagging missing data or a necessary correction before claims are submitted. This reduces the friction often experienced in claims management, where claims must be worked multiple times to gain approval (and payment) from a health plan. When a missing data point or an incorrect code is clearly identified, AI can let the biller know to double-check and correct the claim before it’s sent to the payer. This small improvement makes a big difference. It increases an organization’s first-pass acceptance rate, reduces the rate of claims denials and speeds up the time from creation to collection.
By using AI to proactively offset the risk of denials, advanced technology and human expertise work together to transform the revenue cycle. AI analyzes hundreds of claims in a matter of seconds and identifies those that need attention, which a biller can then focus their time on. In addition to ensuring every detail is accurate in every claim, AI can operate as an overarching “eye” on the revenue cycle. Predictive analytics can be run on all claims to forecast expected revenue collections in a given week or month, helping home health teams benchmark and continuously improve their RCM performance.
Predictive Analytics for a Better Patient Experience
At the patient level, predictive analytics help home health providers see beyond the person in front of them to better understand their clinical and financial needs. These analytics give staff visibility into previous health history and can identify who may benefit from financial assistance—reducing a significant barrier to care for many patients and helping drive equitable access in home health. Predictive analytics can also identify patients who may have difficulty getting to a traditional provider, meaning home health may be a good option to offset a lack of transportation or distance from a provider.
What’s Next for AI in RCM?
While data and AI have emerged as the driving forces for many health care workflows, a human expert will always remain “in the loop,” using AI as a reliable, fast-acting resource to ensure timely care delivery, high-quality care and a smooth revenue cycle.
AI has the potential to reduce many of the common challenges in RCM by arming front-end staff and billing professionals with the real-time, overarching view they need—both to understand trends in their organization’s revenue cycle and to accurately make financial predictions and to quickly address even the smallest (but important) details, such as a missing last name on a claim.
We’re entering an era where many administrative inefficiencies throughout the care journey can be streamlined or augmented with the help of AI to help home health agencies and providers across care settings improve their clinical and financial outcomes.
This AI-powered transformation has already begun and will soon become the norm. Will your organization lead the way, or catch up?